The past decade has been one entirely driven by mass production, consumption, and analysis of data. Businesses now use data-driven decision-making as a core part of their corporate strategies, using data analytics to offer productive decisions and streamline progress. You’d be hard-pressed to find a business that isn’t currently investing in data analytics and big data.
In fact, over 97% of companies are investing in big data projects, with 79% of those stating that they’re worried that other data-driven companies will outperform them. The analysis of data in business intelligence and the success of that business are closely linked, with companies that put data to use being able to make more effective, purposeful, and precise decisions.
Yet, data analytics comes in many different forms. While what initially comes to mind may be purely numerical, quantitative data isn’t the only stream that businesses have access to. Often, by broadening the number of data sources that a company ingests, it is able to develop a more comprehensive understanding of the general market, its competitors, and internal company trends.
In this article, we’ll dive into the world of data analytics, exploring exactly how to improve data-driven decision-making strategies. By exploring each potential data analytics stream, we’ll detail how to put data to work in a way that’s effective, direct, and highly productive. Let’s dive right in.
Types of Data Analytics That Businesses Can Use
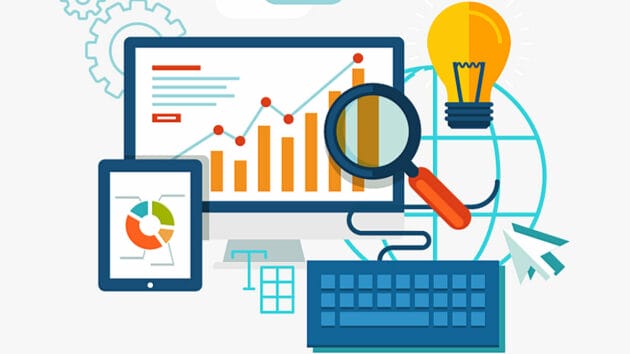
Across an organization, data analytics allows people to get greater insight into processes they come into contact with on a daily basis. Instead of having to make decisions based on opinions or gut feelings, the integration of data analytics provides an evidence-based method of pinpointing the right decisions and executing them.
In 2023, businesses around the globe use data analytics to streamline their processes and optimize every element of their day-to-day operations. You’ll find data analytics everywhere, from marketing and sales departments to HR and more. Especially with the plethora of data democratization tools available to businesses, it’s now easier than ever to create a centralized database where employees can rapidly access the information they need.
Due to how widely used data analytics is, there are a number of different fields of analytics that businesses can choose to focus on. Depending on the nature of a business, the markets they compete in, and its customers, the specific schools of analytics they use may vary. There are four core data analytics systems that businesses use:
- Descriptive Analytics.
- Social Media Analytics.
- Textual Analytics.
- Predictive Analytics.
Let’s break these down further.
Related: How to Use Social Data Analytics to Launch a Successful Marketing Campaign?
1. Descriptive Analytics
Descriptive analytics is the process of analyzing data across a certain period of time to reveal unseen trends, insights, and events. The majority of businesses will have a treasure trove of previous data, spanning their sales records, site visitors, eCommerce data, and more. By collecting this data over extended periods of time, data analytics can draw meaning from it.
Descriptive analytics is especially useful for working out the factors behind certain trends that are happening in a business. For example, if an eCommerce store notices that its sales have suddenly shot up in the last three months, it could use descriptive analytics to pinpoint when this increase began.
By honing in on the specific date, the eCommerce business could align the increase in sales with the day they published their new website design. Past trends allow businesses to answer these “Why” questions, as well as better prepare for the future. If a company notices that sales go up in the summer months, increasing the available stock they have for those months ahead of time will facilitate smooth operations during busy periods.
This form of analytics is one of the most commonly used, mainly due to how broad its application is.
2. Social Media Analytics
As the tools that data analysts have available to them have improved, new fields of analytics have become more available. Social media analytics is a product of this innovation, with businesses around the world now using Natural Language Processing (NLP) to draw meaning from huge amounts of written data.
Social media analytics uses a mixture of NLP and AI to rapidly process huge quantities of data formed by tweets, posts on Facebook, and other social sites. Considering how popular social media has become, this is a fantastic place to learn more about a company’s public perception. By analyzing thousands of posts that mention a brand, social media analytics tools can trace consumer behavior and chart brand sentiment over time.
When a company releases a new product, goes through a rebrand, or simply wants to increase its awareness about how the public perceives them, turning the busy world of social media is an excellent strategy.
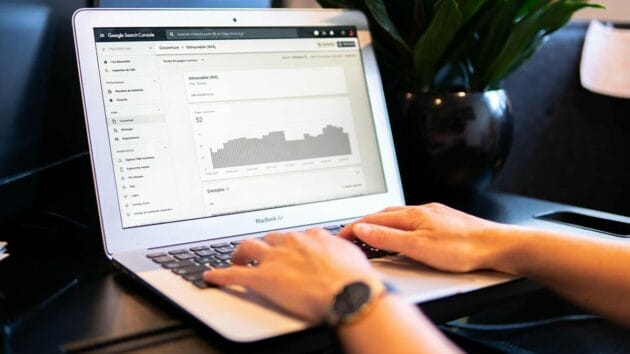
3. Textual Analytics
Textual analytics use many of the same baseline technologies as social media analytics. However, they focus on textual entries like customer reviews, support, ticket, emails, and other text data. Just like with NLP on social media, analysts can ingest data from these textual elements and then run an analysis on them to determine if there are any underlying trends.
Businesses can best use textual analytics to understand consumer behavior and feedback. For example, if a company is running a Voice of the Customer (VOC) program and is collecting feedback, then textual analytics can help to pinpoint what customers want to see improved about a business.
As language processing tools become more effective, textual analytics is quickly becoming a vital tool in the world of customer success, monitoring, and support. By beginning to use textual analytics, businesses are able to digest a huge amount of unstructured data that they can then put to use.
4. Predictive Analytics
Predictive analytics takes historical data and uses it to project a series of likely future outcomes based on past events. If a company has seen a spike in sales in December for the past five years, predictive analytics would suggest that a December spike is likely going to occur again.
Beyond just predicting spikes and declines in sales, predictive analytics can become an extremely precise field. For example, insurance providers will use customer data, claims history, location, age, gender, and other demographic and psychographic factors in order to calculate the relative risk of taking on a new customer.
By estimating the likelihood that a customer is going to claim on their insurance, companies can optimize their pricing strategy to protect their best interests. A secondary field of predictive analytics, prescriptive analytics, is the step beyond this, where companies take action based on the results of predictive analytics.
While past performance does not necessarily guarantee future results, historical data provides a useful perspective that businesses can utilize to optimize their processes.
How to Increase the Efficiency of Data-Driven Decision-Making?
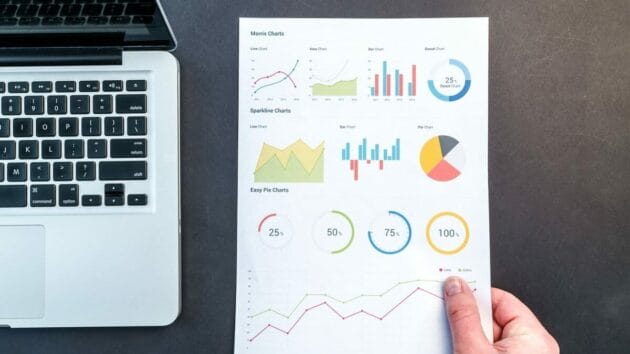
Data analytics doesn’t exist in a vacuum. Each of the methods above can be used in relation to all others. Often, the more information a company has, the better equipped it will be to face anything that the future can throw at it.
With that in mind, there are a number of things that companies can do to increase the efficiency of the handling, processing, and usage of data for decision-making:
1. Streamline Underlying Infrastructure
While quality is important when it comes to data analytics, so is quantity. In order to get the best possible results from analytics, businesses need to make sure that they have as many sources as possible. In order to store all this data and query it, businesses need to make sure they have good underlying data tools. For example, when comparing Apache Pinot vs Druid, one specialized analytics database is more apt for managing and processing data rapidly. In order for your data analytics to perform as well as possible, your business needs to make sure your underlying infrastructure serves the purpose you’re looking for.
2. Create a Data-Driven Culture
Even if your business provides all the data, tools, and visualization structures possible, it won’t become data-driven if your employees don’t understand the power of data. Creating a data-driven culture by demonstrating the importance of using data in the decision-making process is one of the most important steps in becoming data-driven. Once all of your employees understand how and why they should use data analytics, you’ll suddenly see all decisions come from a place of logic, reason, and precise analysis.
3. Centralize Data
While creating a data-driven culture is a powerful way of increasing the prominence of data-driven decisions, this is nearly impossible without data centralization. One of your first priorities should be centralizing data and removing data silos. Once data can flow freely between departments, teams, and sectors of your business, everyone will have access to the knowledge they need to become fully data-driven.
Data analytics is an incredibly powerful asset to businesses, but it only creates transformative change when a company integrates it correctly. By focusing on the underlying infrastructure, company culture, and data management practices, businesses will be ready to step into this data-driven decision-making age.
See also: The Purpose and Methods of Data Collection – Let’s Find Out!
Final Thoughts
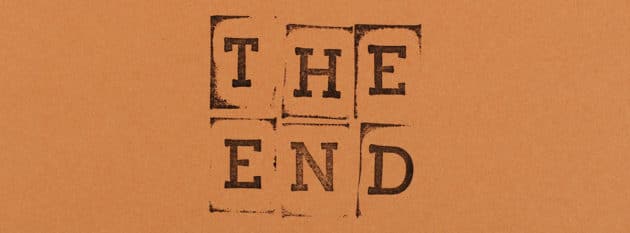
Data-driven decision-making isn’t just a business trend. On the contrary, it’s a central pillar of modern business and one that is driving progress around the world. By incorporating data analytics strategies into daily operations, companies can be more prepared for what the future holds, rapidly optimizing processes, management, and decisions for the best interests of the company.
In order to get the best possible results from incorporating data into decision-making processes, businesses should ensure that they’re properly equipped to handle large-scale data analysis. Focusing on developing the underlying infrastructure to properly collect, transform, store, and analyze data will pay off in dividends in the future.